Word embeddings, trained on large unlabeled corpora are useful for many natural language processing tasks. FastText (Facebook AI) in contrast to Word2vec model accounts for sub-word information. FastText trains embeddings for sub-word sequences of length n (n-grams). For example, instead of only embedding entire sequence “where”, FastText also embed 3-grams: wh, whe, her, ere, re. FastText word representation is the word embedding vector plus sum of n-grams contained in it.
How FastText Works?
FastText embeds words by adding word’s n-grams to the word embedding and then normalizes by total token count:
\( \mathrm{fastText}(\mathrm{word}) = v_{\mathrm{word}} + \sum_{g \in \mathrm{ngrams}(\mathrm{word})} \frac{v_g}{| \mathrm{ngrams} | } \)
If the word is not present in the dictionary, is out of vocabulary (OOV), then \( v_{\mathrm{oovWord}} = 0 \) and only n-grams summed up:
\( \mathrm{fastText}(\mathrm{oovWord}) = \sum_{g \in \mathrm{ngrams}(\mathrm{oovWord})} \frac{v_g}{| \mathrm{ngrams} | } \)
FastText embedding vectors can then be used for word analogy tasks, text classification, or ranking.
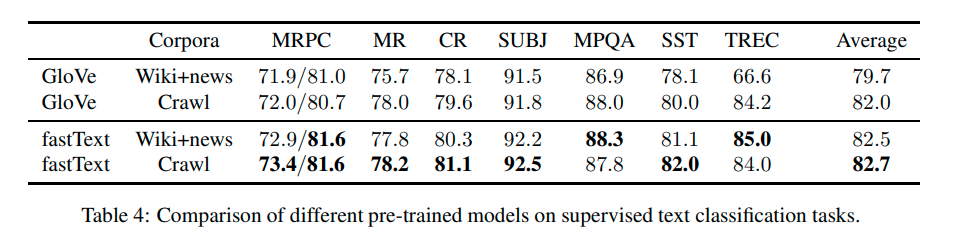
StarSpace vs FastText
StarSpace a general-purpose embeddings is generalization of FastText to objects with some hierarchy e.g. user-content for content recommendation.
Word2vec vs FastText
Word2vec has single word embedding for each word, while FastText has also embeddings for sub-word n-grams and performs the summation above to get the final word embedding. FastText generally outpeforms Word2vec, while having similar requirements.
Embedding Norms
Word2vec Embedding Norms
Word2vec vector norms have been shown (Schakel & Wilson, 2015) to be correlated to word significance. Speculation: If we look on the chart below, then the “middle frequency” words seem to contributing the most, thanks to their large norm, to predict other context words. The very frequent words cannot add much as their appear in too ambiguous contexts. And we don’t have much data about the context of very infrequent words. We can think about the word norms a bit like analogous to TF-IDF, where the IDF is defined on the word2vec window of size 10 words. For this reason when using Word Movers Distance with TF-IDF weights, Word Rotator paper suggests to use consine instead of euclidean distance.
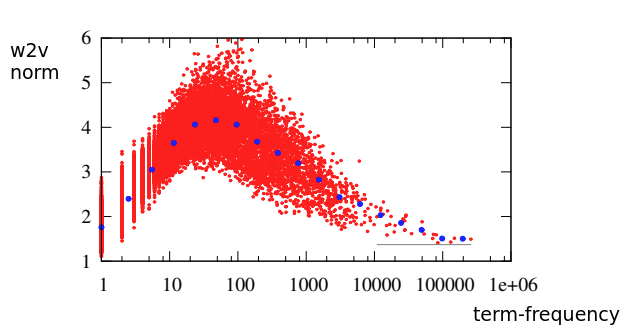
FastText Embedding Norms
How above chart looks in case of FastText? For purpose of studying OOV words this asymmetry between vocabulary and out of vocabulary words is removed by only utilizing word’s n-grams regardless if the word is OOV or not.
In order to study contrast between common english words e.g. “apple” and noise-words (usually some parsing artifacts or unusual tokens with very specific meaning) e.g. “wales-2708” or “G705” MIT 10K Common words dataset is used.
Entire code for this post in available in this repository in file “main.py”. FastText model used is 5-gram English 2M “cc.en.300.bin”.
Standard Vector Norm
Standard vector norm as defined in Gensim implementation is used in this section. Common words are located mostly on the right in the term-frequency spectrum and clustered in three different areas in the norm spectrum. On both axis common words are clustered approximatelly in 4 areas.
From below samples it is not clear what clusters correspond to:
- bottom left cluster: now, three, month, News, Big, picked, votes, signature, Challenge, Short, trick, Lots, 68, priorities, upgrades
- bottom right cluster: our, home, game, won, control, law, common, Street, speed, Tuesday, direct, helped, passed, condition, Date, signed
- middle right cluster: via, companies, necessary, straight, menu, kinds, Championship, relief, periods, Prize, minimal, Rated, 83, wears
- top right cluster: position, wonderful, shooting, switch, â, Atlantic, ladies, vegetables, tourist, HERE, prescription, upgraded, Evil
No N-Gram Norm
As mentioned above each FastText vocab word has its vector representation regardless its size. Norms of those vectors are plotted in this section. The shape of the distribution seems to match closely the shape of the same plot for Word2Vec (Schakel & Wilson, 2015). The vector norm as measure of word significance seems to hold even for FastText in terms of this norm as can be seen from labeled samples in the scatter plot (same frequency bin with increasing vector norm: authors, Alfine, numbertel).
NG_Norm (N-Grams Times Count Norm)
As mentioned above FastText uses average of word vectors used. However for detection of noise-words number of n-grams seems to useful. For that purpose NG_Norm is defined ng_norm(word)= || Σg ∈ ngrams(word)vg ||. Using this norm common words are clustered in narrower band on ng_norm axis.
Explicitly aggregated distribution on ng_norm axis is plotted in histogram below.
Probability distribution of given FastText vocabulary word being common word is plotted below. The distribution is well approximated by t-distribution.
Norms of Hyponyms vs Hypernyms
To evaluate thesis of (Shakel 2015) that word specificity in given term-frequency norm is correlated with vector norm for FastText 67 pairs of hyponyms and hypernyms are used. From just these few examples we see that No-NGram norm with 77% accuracy predicts which word is hyponym and which hypernym disregarding their term-frequencies. Below is the data used. The norm colums contain relative percent differences i.e. (hypo-hyper) / hyper * 100
.
hyper | hypo | standard_norm | no_ngram_norm | ng_norm | count | |
---|---|---|---|---|---|---|
0 | month | January | -22.559890 | 12.179197 | 29.066855 | 76.994548 |
1 | month | February | -34.532857 | 13.354693 | 30.934289 | 57.404248 |
2 | month | March | 21.790121 | 8.177371 | 21.790129 | 91.525721 |
3 | month | April | 25.993046 | 10.371281 | 25.993049 | 86.943093 |
4 | month | May | 247.451639 | 6.607942 | 15.817219 | 219.577850 |
5 | month | June | 86.636376 | 9.665938 | 24.424255 | 80.363607 |
6 | month | July | 93.219042 | 12.777551 | 28.812698 | 71.600872 |
7 | month | August | -4.813989 | 11.601140 | 26.914686 | 56.870358 |
8 | month | September | -44.985682 | 12.394985 | 28.366747 | 61.352990 |
9 | month | October | -21.949212 | 12.073578 | 30.084649 | 64.158556 |
10 | month | November | -35.144106 | 13.222669 | 29.711792 | 55.423498 |
11 | month | December | -34.639645 | 12.905714 | 30.720711 | 59.169547 |
12 | color | red | 214.267874 | -2.442838 | 4.755946 | -14.315463 |
13 | color | blue | 44.778407 | -5.899290 | -3.481073 | -40.683531 |
14 | color | green | -16.087377 | -4.437130 | -16.087392 | -30.029118 |
15 | color | white | -3.950346 | -4.100787 | -3.950355 | 24.457167 |
16 | color | orange | -19.920026 | 1.365383 | 6.773289 | -80.102688 |
17 | color | purple | -25.538990 | -3.007207 | -0.718664 | -87.577665 |
18 | color | black | -5.428290 | -3.726623 | -5.428305 | 26.119314 |
19 | color | pink | 61.684030 | 1.409762 | 7.789344 | -74.939234 |
20 | color | yellow | -25.193438 | 1.552819 | -0.257928 | -71.494422 |
21 | color | cyan | 87.807763 | 18.866982 | 25.205162 | -99.416056 |
22 | color | violet | -28.965518 | 10.081180 | -5.287368 | -98.384650 |
23 | color | grey | 39.793408 | -0.529619 | -6.804404 | -86.867216 |
24 | animal | dog | 252.456093 | -6.000990 | -11.885978 | 76.011544 |
25 | animal | cat | 234.540272 | -6.204253 | -16.364929 | -22.229570 |
26 | animal | bird | 85.873812 | 2.556551 | -7.063093 | -45.238106 |
27 | animal | reptile | -22.501929 | 15.213460 | -3.127411 | -97.982462 |
28 | animal | fish | 75.872201 | 2.111702 | -12.063900 | 22.179526 |
29 | animal | cow | 267.844129 | 7.508819 | -8.038966 | -82.955819 |
30 | animal | insect | -7.264797 | 7.887063 | -7.264797 | -90.300180 |
31 | animal | fly | 259.201598 | -6.115292 | -10.199600 | -24.141623 |
32 | animal | mammal | 3.345599 | 16.280858 | 3.345599 | -96.894255 |
33 | tool | hammer | -60.361552 | 5.061610 | -20.723104 | -88.923340 |
34 | tool | screwdriver | -75.439298 | 33.551341 | 10.523150 | -97.639422 |
35 | tool | drill | -43.531817 | 11.923173 | -15.297724 | -85.132555 |
36 | tool | handsaw | -49.962413 | 76.553452 | 25.093964 | -99.873156 |
37 | tool | knife | -37.435886 | 20.666681 | -6.153829 | -75.100349 |
38 | tool | wrench | -51.094025 | 26.541042 | -2.188051 | -96.230430 |
39 | tool | pliers | -45.382544 | 50.950378 | 9.234910 | -98.404390 |
40 | fruit | banana | -22.010443 | -1.040847 | 3.986077 | -81.245291 |
41 | fruit | apple | -3.168075 | -1.298223 | -3.168080 | -56.612444 |
42 | fruit | pear | 59.400398 | 5.798260 | 6.266932 | -93.565996 |
43 | fruit | peach | -3.104994 | -7.888756 | -3.105001 | -91.252127 |
44 | fruit | orange | -27.728805 | -11.572789 | -3.638405 | -36.933547 |
45 | fruit | pineapple | -55.388695 | 2.253465 | 4.093046 | -91.038789 |
46 | fruit | lemon | 7.380923 | 0.148509 | 7.380918 | -65.358937 |
47 | fruit | pomegranate | -63.004678 | 3.623020 | 10.985970 | -97.139377 |
48 | fruit | grape | 5.267917 | 6.485440 | 5.267921 | -88.986123 |
49 | fruit | strawberries | -65.290713 | 4.979606 | 15.697627 | -88.793171 |
50 | flower | peony | 51.811230 | 15.825447 | 13.858414 | -98.449092 |
51 | flower | rose | 146.126568 | -2.189412 | 23.063286 | 16.749135 |
52 | flower | lily | 108.582103 | 7.221601 | 4.291051 | -93.181922 |
53 | flower | tulip | 49.614036 | 14.132214 | 12.210532 | -96.028388 |
54 | flower | sunflower | -34.754577 | 9.156723 | 14.179479 | -90.751123 |
55 | flower | marigold | -25.294849 | 9.122744 | 12.057728 | -99.084527 |
56 | flower | orchid | 10.703952 | 7.983661 | 10.703952 | -93.583253 |
57 | tree | pine | 9.169017 | 4.525833 | 9.169017 | -86.723162 |
58 | tree | pear | 20.911832 | 11.848664 | 20.911832 | -95.662470 |
59 | tree | maple | -12.181924 | 19.677509 | 31.727102 | -90.921096 |
60 | tree | oak | 155.620050 | 15.579368 | 27.810028 | -85.239221 |
61 | tree | aspen | -16.014889 | 16.366398 | 25.977674 | -99.318727 |
62 | tree | spruce | -32.479379 | 5.101566 | 35.041240 | -97.131057 |
63 | tree | larch | -4.069312 | 22.811006 | 43.896031 | -99.657494 |
64 | tree | linden | -46.235502 | 23.560859 | 7.528996 | -99.731559 |
65 | tree | juniper | -57.948077 | 14.995041 | 5.129804 | -99.002917 |
66 | tree | birch | -20.747948 | 14.309570 | 18.878067 | -97.591876 |
67 | tree | elm | 196.460629 | 20.488973 | 48.230311 | -98.977328 |
68 | average | 25.257317 | 9.337584 | 9.726517 | -44.851693 | |
69 | counts | 42.647059 | 77.941176 | 66.176471 | NaN | |
70 | counts selected | 42.647059 | 77.941176 | 66.176471 | NaN |
Detecting non-english words using NG_Norm
Ability to detect noisy-words is evaluated on simple task of splitting two concatenated words back apart below. For example let’s split back concatenation ‘inflationlithium’:
word1 | word2 | norm1 | norm2 | prob1 | prob2 | prob |
---|---|---|---|---|---|---|
i | nflationlithium | 0 | 4.20137 | 0.000000 | 0.000397 | 0.000000e+00 |
in | flationlithium | 0 | 4.40944 | 0.000000 | 0.000519 | 0.000000e+00 |
inf | lationlithium | 1.88772 | 3.86235 | 0.010414 | 0.000741 | 7.721472e-06 |
infl | ationlithium | 2.29234 | 4.04391 | 0.053977 | 0.000428 | 2.308942e-05 |
infla | tionlithium | 2.24394 | 4.74456 | 0.052467 | 0.000000 | 0.000000e+00 |
inflat | ionlithium | 2.55929 | 3.45802 | 0.048715 | 0.002442 | 1.189513e-04 |
inflati | onlithium | 3.10228 | 3.55187 | 0.007973 | 0.001767 | 1.408828e-05 |
inflatio | nlithium | 3.34667 | 3.26616 | 0.003907 | 0.003159 | 1.234263e-05 |
inflation | lithium | 2.87083 | 2.73886 | 0.017853 | 0.035389 | 6.318213e-04 |
inflationl | ithium | 3.36933 | 2.35156 | 0.002887 | 0.053333 | 1.539945e-04 |
inflationli | thium | 3.73344 | 2.21766 | 0.001283 | 0.052467 | 6.730259e-05 |
inflationlit | hium | 4.16165 | 1.66477 | 0.000096 | 0.004324 | 4.139165e-07 |
inflationlith | ium | 4.40217 | 1.59184 | 0.000519 | 0.002212 | 1.147982e-06 |
inflationlithi | um | 4.71089 | 0 | 0.000000 | 0.000000 | 0.000000e+00 |
inflationlithiu | m | 4.91263 | 0 | 0.000213 | 0.000000 | 0.000000e+00 |
Above approach yielded around 48% accuracy on 3000 random two-word samples from MIT 10k common words. A more efficient method in this specific case would be to search vocabulary instead of calculating vector norms. More appropriate comparison however would be for more general task involving OOV words e.g. using Edit Distance performed also on OOV words and words with typos.
Conclusion
FastText vector norms and their term-frequency were visualized and investigated in this post.
Standard Norm Term-Frequency plot revealed potentially interesting clustering of common vectors in three to four main areas.
No-N-Gram Norm has very similar Norm-TF distribution as Word2Vec shown in (Schakel & Wilson, 2015). The word significance correlation does seem to hold even for FastText embeddings in terms of No-N-Gram Norm.
NG_Norm shows that n-gram count could be potentially useful feature and that simple averaging over n-gram vectors may not be optimal. Perhaps some approach akin to (Zhelezniak et al., 2019) could be used.